Make History with Big Data
Big data refers to the extremely large and complex datasets generated daily by individuals and machines. Imagine the countless messages and photos shared on social media platforms like Instagram or the hours of audio recordings from customer service calls at a national bank. Real-time data from sensors in coffee machines across Starbucks locations or the daily sales transactions processed by Amazon are also prime examples of big data. Hidden within these massive datasets is a treasure trove of insights and valuable information. Through big data analysis, businesses can uncover fascinating patterns that drive innovation and efficiency. In this article, we will dive deep into the world of big data analytics. We’ll explore the five defining characteristics of big data, its benefits, the tools and solutions available, and practical examples. The ultimate question is: how can organizations extract significant value from big data? We will provide actionable steps to help optimize processes, innovate business models, and, like Netflix, make history by harnessing the power of big data. Finally, we’ll share seven practical tips to ensure success.
What is big data?
Big data is data that is unstructured, complex or very large in size. That data is much more difficult to analyze than the simple data that fits neatly into an ordinary database. You need specific tools, techniques, expertise and technology (AI, machine learning, data lakes) to analyze big data. Hence, we define big data as follows:
Big data are large amounts of (unstructured) data that, through thorough analysis, often bring very interesting information and knowledge to the surface. With this you can optimize your business processes and fuel innovation.
This big data definition makes it clear that big data is not just data in a system and you can let it “rest” there. You have to collect it, continuously analyze it, extract value from it and translate that into process optimization or innovative applications. See also the key benefits or order the complete BI book.
The 5 characteristics of big data
Big data can be defined by five key characteristics, all starting with the letter V, making them easier to remember. These characteristics help determine whether a dataset qualifies as big data:
- Volume: The size of the data is so vast or unstructured that it cannot fit into traditional database tables with rows and columns. Instead, big data is stored in modern file systems or object-oriented databases, designed to handle massive amounts of information.
- Velocity: Big data is generated and changes rapidly. For instance, your latest tweet can quickly disappear from followers’ timelines as newer content takes priority. Real-time data from machines and devices is fleeting and can be lost if not captured and processed immediately.
- Variety: Big data comes in diverse forms, including text, audio, video, and sensor data. It also varies in structure and meaning. For example, the word “Washington” could refer to a state, the nation’s capital, or a person’s last name, depending on the context. Even sarcasm in social media posts, such as “Great job, my new laptop crashed on day one,” complicates analysis by adding layers of ambiguity.
- Veracity: Not all big data is reliable. Bots creating fake reviews or spam accounts generating promotional content can distort results. Additionally, incomplete datasets or limitations in APIs can lead to inaccuracies, making it challenging to trust and apply big data effectively.
- Value: The most critical aspect of big data is its ability to deliver actionable insights. Organizations must focus on how big data analytics can create tangible benefits for both the business and its customers. Without a clear value proposition, big data projects risk becoming costly experiments with no return on investment.
Figure 1: To understand the real value of big data, you must first understand the five well-known Vs that characterize big data.
These five characteristics underscore why big data analysis can seem daunting. Research shows that 80-90% of big data projects fail to reach production due to complexity and lack of expertise. Success requires a team of experienced specialists skilled in technology, business processes, analytics, machine learning, and innovation. Instead of searching for a “perfect unicorn,” consider reaching out to our expert big data team to achieve your goals.
What is big data analytics?
Big data analytics refers to the process of collecting, storing, analyzing, and extracting value from big data. This specialized and complex field enables organizations to uncover insights that drive innovation and efficiency. Given the vast size and unstructured nature of big data, traditional methods often fall short, necessitating reliance on advanced technologies such as data lakes for storage and artificial intelligence (AI) and machine learning models for automated analysis. Cloud computing further enhances these tools by providing the scalability needed to process large datasets.
While traditional manual data analysis methods are technically possible, they are typically impractical. For instance, manually analyzing unstructured data can take years and is prone to errors and overlooked insights. Machine learning models, on the other hand, improve accuracy and reliability by training algorithms on massive datasets, enabling better results with each iteration.
The synergy between big data and AI exemplifies a seamless interplay that significantly enhances the likelihood of success. This powerful combination provides organizations with the ability to innovate, make precise decisions, and achieve competitive advantages in a data-driven world.
Types of big data
You may not be taking pictures or recording sound clips yourself, but your organization is likely already generating significant amounts of big data. Consider the log files produced by computers and routers or the massive amounts of data collected from customer interactions. Whether it’s big data or manageable structured data, all storage relies on systems that process information as binary (zeros and ones). At this foundational level, there is no visible difference. However, when analyzed, the following types of big data emerge:
- Documents: examples include emails, quotes, contracts, and text files
- Photos: captured using smartphones, cameras, or specialized equipment
- Videos: recorded with smartphones, video cameras, or advanced systems
- Sound Clips: audio recordings captured through devices like microphones or smartphones
- Sensor or Machine Data: generated by devices, machinery, or other automated systems
- RFID Tags: data from wristbands or chips embedded in products
- Social Media Messages: content created and shared on platforms
- Log Files: generated by computers, websites, and other systems
If you want to start leveraging big data effectively, first identify which types of data are readily available in your organization. Collaborate with representatives from various departments, including data analysts, IT specialists, and business leaders, to brainstorm analyses that can uncover predictive insights. Big data holds immense potential—the key lies in extracting it effectively.
The biggest benefits
Big data analytics may be complex, but when executed successfully, it has a profound positive impact on your organization, customers, and processes. The primary benefits include:
- Streamlined processes: with insights your KPIs can turn green (see also KPIs & Big Data)
- Increased productivity: employees can accomplish much more work in significantly less time
- Higher customer satisfaction: segmentation enables better understanding of your customers
- Proactive operations: shift your organization from reactive to proactive with predictive models
- Enhanced innovation: develop and deliver new products and services faster
- Data-driven decisions: use hard data to guide decisions, complementing intuition
The true power of big data lies in its ability to drive granular, data-driven decisions at the same or even lower costs, following the initial investment.
Figure 2: Some benefits of big data analytics.
Automating big data analysis eliminates randomness and bias from processes. Random sampling becomes a thing of the past as automation enables comprehensive analysis of all cases. This approach also enhances market knowledge, accelerates risk detection, and fortifies your organization financially, making it healthier and more robust in the long term.
Big data analysis: the process in 8 steps
To capitalize on the benefits of big data, follow a structured process and set up an ongoing improvement cycle. These steps ensure your project begins with a clear business issue, which is critical for success. Many big data projects fail to show a return on investment because they are approached purely from a technical or IT perspective. Often, data is collected but not thoroughly analyzed or applied. Below are the eight steps of a successful big data analysis:
Figure 3: The 8 steps of a big data analysis.
- Identify and define the business issue: Collaborate with colleagues to pinpoint which business challenges are suitable for big data analysis. Begin by focusing on the most important Key Performance Indicators (KPIs) in your organization or processes.
- Collect and prepare the relevant data: Based on the identified business issue, select an initial dataset and clean it to ensure relevance and quality. Explore resources on improving data quality to refine this step further.
- Explore and analyze the big data: Use BI tools to explore the data, identify patterns, and assess its potential to address the business issue. Visualize the data in various ways to uncover insights. Learn more about data visualization techniques to maximize effectiveness.
- Compile a final dataset: Repeat steps 1, 2, and 3 until you have a dataset that is complete, clean, and optimized for analysis.
- Build the Big Data Model: Create machine learning models where algorithms can predict, group, or classify outcomes based on the selected datasets for training.
- Validate the model: Have domain experts validate the model’s predictions to ensure accuracy and reliability.
- Bring the model into production: If the model meets the validation criteria and aligns with the business issue, deploy it into production. Ensure data quality remains consistent during this step.
- Evaluate the results of the model: Regularly assess the model’s performance to ensure it continues to deliver accurate results. Use these evaluations to refine and improve the model for greater accuracy.
By following these steps, you ensure a strong focus on solving business problems and establish clear governance with defined roles and responsibilities. This roadmap emphasizes that big data analysis is not a one-time task but a continuous process of refinement and improvement.
Big data solutions & tools
Fortunately, there are numerous big data tools available on the market today. These tools assist in researching and analyzing big data, applying AI, and building machine learning models. According to our annual research (2025) of BI & Analytics solutions, the following software companies are among the top performers in big data: Microsoft, SAS, Oracle, TIBCO, Qlik, SAP.
To explore more, check the BI & Analytics Guide for a comprehensive comparison of these tools.
Big data examples
Learning from the successes of other organizations can provide valuable insights and inspiration. Big data applications are especially prominent in the public sector due to the vast amount of data generated in public spaces. Virtually every area between your home, workplace, and other destinations can generate data for collection and analysis. Here are a few compelling examples of big data applications:
Photos and video images are easy to take automatically these days, for example, by flying drones around with an (infrared) camera. Consider, for example, photos that can indicate whether trees are diseased, gardens are tidy and how high the weeds are. But the photos also show whether parking spaces are occupied by cars without valid permits or indicate the state of maintenance of objects standing in outdoor spaces. Also, there are numerous examples of big data in health care. It is increasingly common to use big data analytics that allow specialists to detect diseases at an early stage.
The BI & Analytics Guide™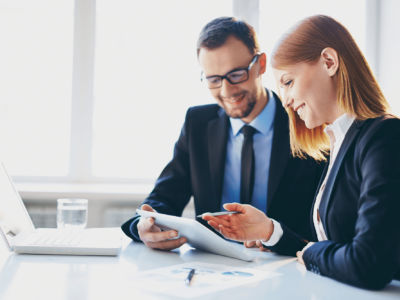
7 tips to achieve success with big data analytics
A successful journey with big data is characterized by an open and learning analytical corporate culture. And, of course, sufficient commitment and budget opportunities from management. In addition, a great deal of business knowledge and thorough process knowledge and creativity is required from both the business people and the data scientist. To achieve success with big data, a project leader further ensures:
- Alignment with the organizational goals and KPIs: The big data goals align with the strategic goals and KPIs, so that big data can make a substantial contribution to achieving the organizational goals. Just building a data lake at random is pretty useless. Make sure not the technology is leading, but your business strategy, KPIs and business processes.
- Involved users: User participation and especially user awareness of what big data can mean for their own work process is of great importance for the success of a project. An agile & scrum approach can help realize that participation. But a training course on big data or an inspiration session should not be missing either.
- Source and data quality: Data quality is of even greater importance with big data than with normal data analysis that you usually apply with Business Intelligence. After all, with big data you are going to make certain decisions automatically. The data tells you to turn left now instead of right. A machine learning model put into production often functions as a black box. Furthermore, in a data lake, there are still hardly any facilities available to measure and improve data quality across the board.
- Ethics & privacy: When it comes to the processing and analysis of personal data, laws and regulations such as the General Data Protection Regulation (GDPR), the California Consumer Privacy Act (CCPA), or the more recent AI Act can quickly put up quite a block to successfully applying big data analytics.
- A solid data foundation: The quality and flexibility of the data infrastructure must also be high. You need a robust and scalable system because photos, texts, machine data, and video images can soon require petabytes of storage. Although storage space doesn’t cost that much these days, size remains a concern. Also, big data analysis can quickly get bogged down by the complexity of the data. So you need a lot of “brute” and also smart computing power to set up a good system with which to develop an application quickly and agilely.
- A balanced team structure: There are enough experienced data science experts and a team in which you can align business and IT skills and competencies well. That way you can respond better and faster to various information needs and complement each other.
- Usability and ease of use: Finally, the usability, accessibility, and ease of use of a big data application must be high.
Managing the above issues well and achieving success with big data analytics is by no means an easy task. They interact with each other and require a steady hand, solid expertise, and a good dose of experience with big data analytics. Request no-obligation consulting here.
Learn more about big data analytics here
To facilitate you even better with knowledge and best practices about the field of big data analytics, we provide an overview here for more depth:
If you yourself don’t understand big data well (enough) then you risk failure. See our 7 tips for success.
Getting started with big data?
Do you want to start with big data analytics? Feel free to contact us for an exploratory discussion with one of our big data specialists. We would love to help you get your organization working data-driven and we get very happy when we can help you with successful big data applications.
About Passionned Group
Passionned Group is the specialist in designing and implementing innovative big data solutions. Our passionate big data consultants help companies and governments in their transition to an intelligent, data-driven organization. Every other year we organize the Dutch BI & Data Science Award™, the election of the Smartest organization in the Netherlands.
Frequently Asked Questions
Why should you get into big data analytics?
First, because you probably already own a lot of big data without perhaps even knowing it yourself. But more importantly, big data analytics allows you to discover patterns that can greatly accelerate and improve your processes. Get inspired by the examples or check out our Data Science book.
How do I ensure secure processing of my big data?
You need to comply with privacy and other laws to ensure the secure and ethical processing of data. In the United States, laws like the California Consumer Privacy Act (CCPA) and sector-specific regulations such as HIPAA for healthcare data play a critical role. These frameworks aim to protect individuals’ data from misuse and ensure transparency in data handling. Additionally, emerging regulations, including guidelines for artificial intelligence, are likely to expand protections for users of big data and AI technologies.
What do the 5 Vs that characterize big data mean?
Volume, Velocity, Variety, Veracity and Value. These are the five characteristics by which you can recognize big data.
What are the main big data platforms?
The best-known big data platform is Hadoop. Also popular are Apache Spark, Apache Cassandra, MongoDB, Apache Kafka, Microsoft Azure HDInsight, Google Cloud BigQuery, Amazon’s Elastic MapReduce, Cloudera and IBM InfoSphere BigInsights. For a comparison of different big data platforms, consult the BI & Analytics Guide 2025.
What roles do I need in my project?
First and foremost, a business consultant who has successfully executed big data projects before. In addition, you need a data analyst, a data scientist or machine learning engineer and a data engineer. Contact us for hiring.
What is not big data?
To determine whether data qualifies as big data, consider whether it fits neatly into a structured table of rows and columns. If the answer is “yes,” it likely does not fall under the category of big data. However, when the data exceeds the constraints of traditional tables—either due to sheer volume or a lack of structure—it becomes what we define as big data.
What does zero data mean?
Zero data is data that is not stored as such but that you can still retrieve using techniques such as inference.
How can you measure data quality?
You can assess the quality of your data using a number of criteria such as completeness, accuracy, uniqueness, validity, timeliness and consistency.
Is there a difference between big data and open data?
Yes, open data is information that is freely available to the public without restrictions on access or use, allowing any citizen or organization to access, reuse and share it. Big data can also be made available as open data.