And now for something completely different: Data Mesh
The term data mesh is popping up more and more often. Few, however, can explain exactly what it is and what it stands for. If you talk to data engineers and architects about the data mesh, data pipelines, connectors, controllers, and processors soon dominate the conversation. That’s nice if you’re a “techie”, but as a business manager, you want to be able to make sense of things and see the complete picture in front of you. And as an architect, you want to understand how a data mesh fits into the enterprise architecture. Multinational companies like Netflix, Zalando, VistaPrint, and Intuit are enthusiastic about it. But what does this philosophy entail, and isn’t data mesh just another hype? We wanted to find out and asked the experts Rick van der Linden and Daan van Beek of Passionned Group.
What is a data mesh?
Central or decentralized, that is the question. ‘A data mesh is an architecture where you can build your Business Intelligence locally, and decentralized at the domain level. You package the results as a full-quality product. You’re going to deliver that product to customers in other business domains. This includes both transactional and analytical data’, Van der Linden explains.
Figure 1: A simplified view of a data mesh architecture
A quality product, that sounds nice. But to immediately clear up a misunderstanding, a data mesh is not a concrete product, according to Van der Linden. Nor is a data mesh a special software tool that you can order off the shelf from a supplier. Although some vendors would have you believe otherwise.
Where does data mesh come from?
According to the creator of the concept, Zhamak Dehghani, former principal consultant and director of technology company Thoughtworks, a data mesh is a decentralized data architecture where business domains own their data. They themselves are responsible for the access, storage, performance, quality, and delivery of data products. They autonomously – in a federated business model – monitor data quality, and security and provide data governance. Data is made available for both transactional and analytical purposes (Business Intelligence).
Based on a self-service model, the various business domains can request the necessary data from the other domains. So, not from a central Business Intelligence department anymore. Why would you still want to copy and move data so far away from the primary process? Governance is taken care of by working together in a federated business model.
That doesn’t happen automatically, according to Van der Linden. ‘You can’t organize that quickly. Just like in the “real world” a federation involves a lot of coordination, communication, and politics. Think for example of the United States, the Swiss cantons, or the Federal Republic of Germany. Nevertheless, federation is a popular form of organization. Even in a data mesh, you have to get everyone on board.’ Dehghani says she developed the data mesh as a third-generation data platform, an alternative for organizations that get stuck with the precursors of the data mesh, such as a central data warehouse and a data lake.
Data mesh tackles poor data quality at the source
Van der Linden says: ‘A data mesh is a new promising philosophy, an alternative approach, a different mindset for dealing with data. It is perhaps less concrete than a data lake where you can have a cluster of servers on which you dump all your data.’
A data mesh offers a solution to the poor data quality that organizations have been struggling with for decades.
‘In my view, a data mesh finally offers a solution to the problem of poor data quality that organizations have been struggling with for decades. After all, I don’t believe in a central department monitoring data quality. It too often leads to discussions: which data source is leading and why are there two versions of the truth each time?’
A Data mesh requires strict governance
Van der Linden continues his plea for a data mesh: ‘If you are a data manager within the Human Resources business domain, for example, then you are responsible for the data quality of all personnel data. You will have to ask yourself the following questions: is the data correct and are you able to offer data as a product in a decent way to everyone in the organization who needs it for their work?’
‘If everyone adheres to the agreements regarding metadata, definitions, delivery specifications, communication protocols, and so on, within a data mesh it can no longer happen, for example, that due to all kinds of copy runs and timing differences, “the number of access control badges issued” does not correspond to “the number of staff employed”. To give just one small example of a quality issue. This example still involves the delivery of one piece of data, but even with bulk data, you must be able to blindly trust the information delivered. After all, PZ is closest to the data source and is the most logical party to guarantee the data quality of personnel data.’
The Data Science book for Decision Makers & Data Professionals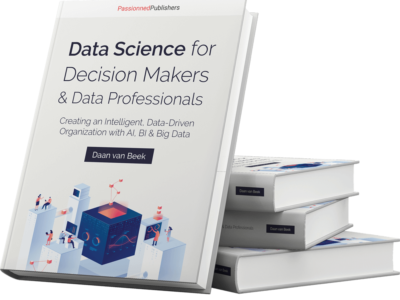
Data mesh bridges the gap between operational data and analytical data
In her seminal articles on the potential of a data mesh, Dehghani identifies several more bottlenecks in current enterprise architecture that Van der Linden is happy to comment on. For example, a gap has developed over time between operational (transactional) data and analytical data. The analytical discipline has different requirements. Retrieval of data is often not done by keys but by attributes. Also, it always involves large amounts of data at once, rather than messaging. Because data mesh dictates that both transactional and analytics are produced locally (in the same place), that gap disappears.
Data mesh simplifies plumbing
Moreover, the gap has led to a fragile architecture. ETL (Extract, Transform, Load) tasks stagnate as window times get longer and longer due to excessive data volumes, a huge amount of data sources, hardware overload, unfamiliarity with the (in)capabilities of the ETL Tools used, and so on. This increasing complexity has created a labyrinth of data pipelines. Pipelines that architects are trying to connect, with data flowing from the operational data plane to the analytical plane, and back to the operational plane. The data mesh reduces complexity by dividing the labyrinth into planes. In it, each domain picks up its own tasks.
Van der Linden: ‘So, when we talk about architecture, we’re talking flatly about the most efficient way you’re going to weld data together with pipes via connectors, controllers, and processors. So, plumbing in the context of a corporate network, and that’s not sexy at all and almost never produces immediate results.’ But for large companies like Netflix, and Zalando, which must process trillions of events and petabytes of data every day, it is vital. Yet data mesh is not only a solution for these multinationals. Van der Linden notices that medium-sized and large Dutch municipalities, with a multitude of domains, are also showing interest because they face similar problems. In an illuminating video, Justin Cunningham of Netflix explains how a data mesh can significantly simplify plumbing.
From push to pull
In short: a shift in our thinking is needed, a paradigm shift as Dehghani calls it. This will allow you to address the bottlenecks mentioned above. The traditional push model where large amounts of data are pushed through ETL pipelines will have to give way to a pull model where business domains deliver data products just like consumer products. ‘Data packaged in a package, not a semi-finished product, but an integral product with a nice bow around it’, Van der Linden explains evocatively.
The four principles of a data mesh
According to Dehghani, the four principles below, when interrelated, give the data mesh meaning and substance:
Principle 1: Choose domain ownership
Instead of letting domain data flow to a central data lake or platform, domains should now host and offer their domain datasets themselves in an easily consumable way.
Decentralize ownership of transactional and analytic data to business domains that are closest to the data. Organize it so that those domains control the source of the data or are its main consumers.
Principle 2: Data-as-a-Product is leading
Approach the transactional and analytical data from the domains as a product (Data-as-a-Product) and treat the consumers of that data as customers and as “happy and delighted customers”, as Dehghani euphorically calls them. Customers, such as data users, data analysts, data scientists, and so on, are allowed within a data mesh to make demands on the quality, accessibility, discoverability, interoperability, security, understandability, reliability and integrity of the data.
Important message from Zhamak Dehghani:
Customers are allowed to make demands within data mesh regarding data quality, data reliability, and data integrity.
Principle 3: Build a self-service platform for data
Create a self-service data platform that enables domain teams to share data. The platform is aimed at removing friction throughout the data-sharing journey, from source to use. Data platform services manage the full lifecycle of all individual data products.
Principle 4: Adopt federated control and governance
Establish an operational model for data management based on a federated decision-making and accountability structure. Establish a team consisting of domain representatives, controllers, and experts in legal, compliance, security, and so on.
The federated business model creates an incentive and accountability structure that balances the autonomy and agility of domains with the overall interoperability of the network. That is, despite domain autonomy, all people and systems can work together without constraint. And that no one domain can become obstructive because, for example, their employees do not want to share their data.
Learn more about data warehousing
Is data mesh knocking out the central BI department?
Daan van Beek: ‘When I let these four principles of data mesh sink in, I see the potential of this new philosophy on the one hand and the enormous impact on the organization and enterprise architecture on the other. As I see it now, this could well be the death knell for the central BI club as we know it today and with which I have grown up over the past decades. Consistently applying the principles of a data mesh basically means dismantling your central BI department. In addition, you’re also going to incur additional costs initially.’
Data mesh could well be the death knell for the central BI department as we’ve known it until now.
‘Each individual business domain, for example, must also have access to a powerful data warehouse and servers. Another issue is that some of the carefully accumulated centrally pooled BI knowledge will be lost. Organizations that go for a data mesh must be well aware of all that. In short: a data mesh is a difficult but passable path. Zalando had also initially set up a central data warehouse shortly after its founding in 2008. But never forget: a bird in the hand is worth two in the bush.
An interim assessment: differences between data warehouse and data mesh
Let’s take some time to reflect. To organize and sharpen our thoughts, we list the main differences between a central data warehouse (team) and the data mesh architecture in the adjacent table.
Table 1: Comparison between Central Data Warehouse and Data mesh
Data mesh: a tricky business?
So, there are quite a few drawbacks to the data mesh, according to Van Beek. It means a big change in the setup of BI & Analytics. Each business domain is going to get its own (small) data warehouse (marketing, sales, production, HR, finance, et cetera) with all its consequences. In addition, you still need a central BI architecture for analytics that is cross-domain, either through a central data warehouse or using data virtualization. Because usually, meaningful analytics requires combined data from different domains. Consider productivity per employee. The central self-service platform (principle 3) makes this possible. But of course, you have to organize and manage this centrally.
Data mesh reduces complexity
Van Beek: ‘What problem do you actually solve with a data mesh? For example, to monitor and improve data quality, we now have data stewards sitting in the domains. My experience is that BI, wherever you organize it, is technically complex and involves real engineering work. I think the biggest challenge is delivering analytics products that allow domains to improve their decisions in the operational process. But for large or more complex organizations (multinationals, municipalities) and data warehouses, there is a limit to the capabilities of a central model. You can then reduce the complexity with a data mesh architecture. In essence, we don’t necessarily need a data mesh, a data warehouse, or a data lake, but we do need BI teams that get very close to the business and will develop and use business intelligence together with the business.’
Five arguments in favor of a data mesh
Partly at the request of several Passionned Group customers, Van der Linden delved into the unprecedented possibilities offered by a data mesh. As a result, he came up with five arguments in favor of a data mesh. Briefly, they are as follows:
1. A data mesh is based on sociotechnical principles that make sense
A data mesh is usually mentioned in the same breath as sociotechnics, a business movement aimed at improving the functioning of people and organizations by adapting or redesigning organizations, work processes, and human work tasks. Sociotechnology focuses on the interactions between people and the technical architecture and solutions in complex organizations. With principles such as self-direction and integrated management, a focus on value addition, teamwork, horizontal coordination, and decision-making based on partnership and dialogue, sociotechnics is incidentally on the same wavelength as a data mesh and the intelligent, data-driven organization. Therefore, embrace the sociotechnical approach and act on its principles.
2. A data mesh finally gives data the status it deserves
Data is a commodity in its own right. How do you extract as much value as possible from your data? According to McKinsey, there is only one way: manage it as a product. Otherwise, you’re not going to make it through today’s data explosion. The key is to manage and package data as you would a consumer product. And this very call aligns with the principles of a data mesh. This does mean freeing up people and resources.
Every data product should have a product manager and a team consisting of data engineers, data architects, data modelers, data platform engineers, marketers, and so on. In addition, standards and best practices are indispensable. You will need to measure the performance of data product teams and establish a quality assurance system. To confirm data integrity, product data teams work closely with data stewards who own data source systems. So, don’t treat data merely as a byproduct of digital transactions; instead, put data at the center, as a product in its own right.
3. Business domains are much better able to assess data quality
Until recently, the emphasis within a centralized data architecture was still on the certification of the so-called “gold datasets.” These were the datasets that had gone through a centralized process of quality control and certification and were deemed reliable. Within the data mesh architecture, this process has become obsolete. It no longer requires a centralized team and management group to clean up, harmonize, and code the data. A data mesh completely decentralizes these tasks. A domain dataset becomes a data product only after it has gone through the process of quality assurance locally, within the domain, according to the expected quality criteria and standardization rules. Indeed, domain data owners are best equipped to decide how to measure the quality of their domain’s data because they know the details of the domain operations that produce the data. Therefore, aim for 100% data quality at the decentralized domain level.
4. A data mesh paves the way for data virtualization
Data virtualization is the integration of data from multiple data sources, without physically replicating the data. As a user of the data, this means you only need to address one data source. Data virtualization therefore involves multiple sources coming together in a single, abstract virtual layer. In fact, to the end user, it is invisible where the data is stored. Several companies and municipalities are already modernizing their BI landscape using data virtualization. Data virtualization allows them to make large amounts of data accessible in an unambiguous way, without moving or copying the data to a central location. So, the user uses the source data directly, not copies. This ensures that you are always using the most up-to-date data. Another advantage of data virtualization is that users do not have to have technical knowledge of the underlying data sources. So-called intelligent data connectors establish a connection to the source systems. Therefore, like the Dutch municipality Gemeente Sittard-Geleen, immerse yourself in the obvious advantages of data virtualization within the context of a data mesh.
5. Data mesh and Common Ground principles complement each other perfectly
It is not only large multinationals such as Netflix that reap the benefits of a data mesh. Government agencies, such as medium and large municipalities, also struggle with large amounts of data that they continuously collect, store, manage, and access per domain in endless different administrations. Examples include housing and parking permits, issued passports and driving licenses, fees to be collected, as well as the Personal Records Database (BRP) and Land Registry data hosted on external servers. Large municipalities are also expected to maintain many hundreds, in an extreme case as many as 1,200, different applications on-premise or in the cloud.
Preparing for data mesh
With the emergence of the Dutch Common Ground movement, and associated redesign of information services, some municipalities have already pre-sorted for a data mesh. After all, one of the central tenets of this Common Ground movement is that data is stored only once. Thus, data is decoupled from work processes and applications. It follows the principle of loosely coupled highly aligned. Data is now retrieved at the source, instead of being copied and stored frequently. No more copying data from the BRP every night. The data stays nicely where it belongs, behind firewalls. That enables municipalities to respond to social issues in a flexible way. The really ambitious municipalities are therefore not only joining the municipal Common Ground movement but are also seriously studying data mesh and data virtualization.
Consequences for the enterprise architecture
Clearly, the application of data mesh principles in combination with data virtualization has implications for the ideal data warehouse architecture and the “architectural picture” of the intelligent, data-driven organization. The databases and BI knowledge are now concentrated close to the source by business domain. In effect, all new, small silos are created. But unlike the old, damned data silos, in which the inconsistent, fragmented, and often duplicate data got stuck and became more and more isolated and alienated from the rest of the organization, with the data mesh the information is accessible to everyone in the form of Data-as-a-Product. The data virtualization layer provides the enterprise BI.
Data mesh is less abstract than you think
Despite the somewhat abstract nature of a data mesh and the still relative unfamiliarity of the phenomenon, Van der Linden expects that data mesh could develop into a basic philosophy, the prelude to a fully-fledged enterprise data architecture. ‘If you take data quality seriously, you will have to set up an architecture that does justice to all the interests of all stakeholders and at the same time lets the stagnant data flow again. Wise CIOs and curious IT managers are therefore now delving into data mesh theory and the practical examples available.’ Contact Rick van der Linden now for initial orientation.
Final thoughts
Organizations keep searching for the most effective and efficient way to manage, structure, analyze, and make usable large amounts of structured, semi-structured, and unstructured data. We now have a range of modern techniques and concepts. How do you best deploy an analytical database, a data warehouse, a data mart, a data lake, a data lakehouse, a data hub, a data fabric, or a data mesh? That’s the challenge. In any case, make sure the choice is deliberate.
Conclusion
A data mesh addresses many of the pain points organizations are struggling with today. And the data mesh can accelerate organizations’ ambitions to become more data-driven. A data mesh is technology-neutral and provides a common language. It is an interesting alternative to the data lakes and centralized data warehouses that organizations have established on a large scale. But remember that with the data mesh, you will turn everything upside down. Moreover, you will have to jettison the (architecture) choices of the past.