Enhance decisions using data and insights
In today’s fast-paced world, decision-making is a constant, whether it’s choosing your next vacation or determining when your organization should adopt AI. Often, we rely on familiar methods because they’ve worked in the past, but it’s important to reflect on why we make decisions the way we do and whether better alternatives exist. Making smarter decisions involves understanding the decision-making process, considering new approaches, and using data to guide our choices. On this page, we’ll explore these aspects in depth—from the decision process to making clear, well-considered choices, and how to make data work for you—so you can make more informed decisions that lead to greater personal and professional success.
What is a decision?
Understanding exactly what a decision is can be challenging. We often say a decision is a choice, but what does that really mean? After all, making a choice is just one part of the decision-making process. We make decisions all day long, so it seems natural to assume we know what a decision is.
Simply put, a decision is the confirmation of a choice.
However, we’re not always aware that we have a choice. Every action you take can be seen as a decision because you can choose to do it or not. This means any choice can have unintended consequences, much like the butterfly effect, where the small action of a butterfly flapping its wings could potentially lead to a hurricane. On the other hand, a choice you’ve agonized over might have little impact in the end. It can be helpful to dissect your decisions, as you gain a deeper understanding of what it means to make a particular choice. While perfect options are rare, it’s reassuring when you feel confident that the decision you made is the best one possible.
Herbert A. Simon’s Decision-Making Theory
In his monumental book “Administrative Behavior”, Herbert A. Simon was one of the first organizational scientists to describe how decisions are made within organizations. He explains that while organizations often have clear goals and missions, translating these abstract intentions into consistent actions can be challenging. Research shows that in as many as 78% of organizations, the mission and goals are not fully integrated into day-to-day operations. When making a decision, an organization must consider how well a given choice aligns with its intended goals. However, there are often multiple options that seem to contribute to these goals but may be mutually contradictory. For example, a town hall might need to choose between investing in more green space or building additional roads in a neighborhood. Both options could enhance livability, but the town hall cannot implement both. So, how do you arrive at the right decision?
Simon describes how a decision should theoretically come about in three steps:
- Identify all available choices.
- Estimate the consequences of these choices.
- Compare the different consequences to find the best choice.
Figure 1: The first step is to identify all the different choices, the second step is to assess all the consequences of your choice, and the third step is to compare the different consequences to find the best choice.
This is, of course, an idealized view of decision-making, and Simon is well aware of this. In reality, it’s nearly impossible to identify all potential choices, as the possibilities can be infinite depending on the decision at hand. The same challenge arises when estimating the consequences of a decision. While it’s possible to predict some effects, you can never gather all relevant information. This is due to the sheer volume of data, which is constantly changing and can influence your decisions in unforeseen ways.
Bounded rationality
But how does one actually make a decision? Simon uses this approach to critique the concept of the “homo economicus,” which assumes that people always strive for the best possible outcome because it is the most rational choice. Simon argues that this is unrealistic, as making the best choice would require considering an endless amount of information—a nearly impossible task. Instead, Simon introduces the concept of “bounded rationality,” which suggests that the rationality behind decisions is limited by various constraints. The way a decision is made depends on the decision-makers perspectives and the specific context they find themselves in.
Since it’s impossible to account for every factor that influences a decision, decision-makers must rely on a simplified version of the world. This means the decision-making process described earlier remains relevant, but with the understanding that not everything can be included. While the homo economicus model views every choice as an exercise in maximization, bounded rationality suggests that the best choice is often the most feasible one. However, biases and noise also play crucial roles in this process.
Simon’s theory, developed in the 1940s, remains relevant today. Bounded rationality provides a realistic portrayal of how decisions, both personal and organizational, are made. We can rarely make the most “rational” decision in an absolute sense. If we knew exactly what the best decision was, there would be no doubt. In practice, we usually make the decision that seems most acceptable given our constraints. Therefore, while Simon’s decision model acknowledges that making the “perfect decision” is impossible, it still offers valuable guidance by encouraging us to strive for the best possible outcome within our limitations.
Data-driven decisions
A data-driven decision is one that relies heavily on concrete data and facts. While organizations today gather vast amounts of data, this information is not always effectively utilized to enhance decision-making. Often, the challenge lies in understanding how to best leverage this data. One effective approach is to use the data-driven PDCA cycle. PDCA stands for Plan, Do, Check, Act. This method involves developing a plan, executing it, evaluating the outcomes, and then refining your approach based on the findings to improve your working methods.
Figure 2: This figure shows how the PDCA cycle applies to decision-making. It emphasizes that improvement is continuous, with the cycle never truly ending as you constantly iterate through Plan, Do, Check, and Act.
You start with information from (big) data, combine it with past experiences to form knowledge and make a decision based on this. The resulting action ideally produces an outcome that is reflected back into the data, allowing for further reflection and analysis. This process enhances your experience, leading to better future decisions.
By following this cycle, organizations can continuously refine their processes and leverage data more effectively. Planning clarifies goals and methods, execution gathers data, and monitoring assesses the plan’s effectiveness. Insights from this analysis are then used to optimize future plans, fostering improved, data-driven decision-making.
White paper ‘The PDCA cycle is alive and well’
Tools for data-driven decisions
To make smarter decisions using data, you can leverage Business Intelligence (BI) tools. These tools offer various features and benefits, each with its strengths and weaknesses. We’ve developed a comparison tool to help you evaluate different BI options and identify the one that best fits your organization’s needs. This resource simplifies the process of finding the most suitable BI tool for your specific requirements.
Case Study: Cape Town’s data-driven COVID-19 decision
During the COVID-19 pandemic, the City of Cape Town in South Africa implemented a data-driven approach to manage the crisis effectively. The city’s Policy and Strategy Department, in collaboration with various research institutions, used data analytics to deal with several key challenges posed by the pandemic.
They focused on quantifying the costs of COVID-19 initiatives, managing a rise in fatalities, and optimizing the allocation of scarce public resources. By analyzing real-time data, the city could make informed decisions quickly, enhancing their ability to respond to the evolving crisis. For instance, data on infection rates and resource availability helped in dynamically adjusting healthcare and emergency services to areas with the highest need. This approach significantly improved the efficiency and effectiveness of Cape Town’s response to the pandemic, highlighting the critical role of data in managing public health emergencies.
Benefits of data-driven decisions
Curious about how data-driven decisions can benefit your organization? Here are some key advantages:
- Increased Accuracy: Decisions based on data and facts are a more precise reflection of reality compared to those based solely on intuition or feelings.
- Reduced Risk: Data-driven decisions are less dependent on intuition, minimizing the risk of errors and enhancing objectivity.
- Improved Efficiency: Real-time data enables automation, such as algorithmically setting product prices, which boosts productivity and streamlines decision-making.
- Improved Predictability: Analyzing historical data helps identify trends and forecast future scenarios, preparing organizations for upcoming challenges.
- Consistency in Decisions: Data-driven decisions apply uniform standards and metrics, leading to more consistent and transparent decision-making.
- Adaptability: Data provides insights into market changes, facilitating agile responses and effective adjustments to evolving conditions.
Figure 3: The benefits of data-driven decisions: increased accuracy, reduced risk, improved efficiency, improved predictability, consistency in decisions, and adaptability.
Creating a decision tree
A decision tree is a valuable tool for visualizing decisions. You start with a central point and create branches for each possible choice. From each choice, further branches represent the potential outcomes (see illustration below).
Decision trees can be used in various ways. While they are commonly employed in developing algorithms for Artificial Intelligence, they are also effective for defining rules in repeatable situations. In these cases, branches are determined by specific conditions rather than probabilities.
Figure 4: Example of a decision tree: what does the municipality need to know and consider before embarking on a project?
Reducing bias and noise for better decisions
What is a “pure” decision? A pure decision is one made without unintentional influences such as biases and noise, recognizing that human rationality is not perfect. Many factors can affect decisions beyond rationality, making it crucial to be aware of these potential contaminations. For insights on minimizing these influences and achieving clearer decision-making, read our blog, “The 20 Best Tips for Pure Decision-Making.”
What is the concept of noise?
The book “Noise,” by Olivier Sibony, Cass R. Sunstein, and Nobel laureate Daniel Kahneman, explores how bias and noise impact decision-making. While bias—systematic errors due to flawed reasoning—is relatively well-understood, “noise” refers to inconsistencies in decisions that should be identical.
For example, in the legal system, bias is evident when individuals from immigrant backgrounds receive harsher sentences for the same crimes as those from native backgrounds, reflecting systemic unfairness. Conversely, noise is observed when different judges, faced with identical facts and laws, render varying judgments on the same case. This variability arises despite having the same information and legal standards, highlighting that noise distorts decision-making.
Noise demonstrates that our choices are never entirely objective, though it is possible to make less subjective decisions.
Bias introduces predictable distortions, while noise causes unpredictable variations. Both undermine decision reliability, underscoring the need for striving toward more objective and consistent decision-making.
Tips for smart decision-making with less noise
Like bias, noise can be addressed through various strategies. Awareness of noise is crucial, as detailed in the book “Noise.” Decision-making involves prediction—anticipating how a decision will impact the future—and judgment—evaluating how it aligns with past decisions. Both aspects can be affected by noise, but implementing good decision hygiene can help mitigate its effects. Here are some tips from the book:
- Standardize the Decision Process: Identify key factors in recurring decisions and use checklists or standardized procedures to ensure consistency. Refer to our decision chart for guidance.
- Training and Education: Educate your employees on recognizing and mitigating cognitive biases and noise, so they can make more accurate decisions.
- Use of Data and Data Analysis: Leverage data to enhance objectivity. Data visualization can highlight patterns and trends that might be overlooked with raw numbers alone.
- Group Decisions and Diversity: Incorporate diverse perspectives and collaborative decision-making to reduce bias and enhance decision robustness.
Applying these strategies can help minimize noise and lead to more effective and equitable decision-making within your organization.
The Pros and Cons of Group Decision-Making
Making decisions in a group context has several advantages, including the integration of diverse perspectives and fostering broader acceptance of decisions. When decisions are made collaboratively, participants are more likely to commit to and understand the rationale behind the decision, leading to more consistent implementation. Involving stakeholders also allows for concerns to be raised and addressed, which can strengthen support for the outcome.
However, group decision-making also has pitfalls. Social dynamics can lead to conformity, where individuals may suppress their doubts or concerns to avoid disrupting group cohesion. This can result in less critical evaluation of options and even groupthink, where the desire for consensus overrides thorough analysis. Therefore, it’s crucial to be mindful of the influence of noise and biases, even in group settings, to ensure that decisions remain objective and well-informed.
Methods for group decision-making
Here are some methods to facilitate group decision-making:
- Brainstorming: Discussing a concept or problem together can reveal diverse perspectives. Creating a mind map, as illustrated below, fosters creativity and enriches the discussion
- Delphi method: Named after the Ancient Oracle of Delphi, this approach involves gathering opinions from multiple experts independently. Their feedback is then aggregated and shared back with the experts, providing high-quality insights for decision-making.
- Consensus decision-making: A decision is only made when all group members find it acceptable, even if they don’t all fully support it. This method often leads to better buy-in and reduces strong objections compared to decisions made without consensus.
Figure 5: Collaborative decision-making can occur at different stages of the process: during the exploration of choices (brainstorming), the evaluation of options (Delphi method), and the final decision-making (consensus decision-making).
The role of time in decision-making
Time is a crucial factor in decision-making because the availability of information and the complexity of situations evolve over time. Taking sufficient time allows you to gather more data and consider the potential consequences thoroughly, leading to more informed decisions. However, excessive deliberation can lead to indecision and missed opportunities. Striking a balance between careful analysis and timely action is key to effective decision-making.
Additionally, the timing of decisions significantly impacts their outcomes. Strategically timed decisions, such as launching a product at an opportune moment, can provide a competitive edge. Proper timing also helps manage risk and reduce negative consequences. By making decisions in stages and regularly evaluating them, you can adapt more effectively to new information and changing circumstances.
The BI & Analytics Guide™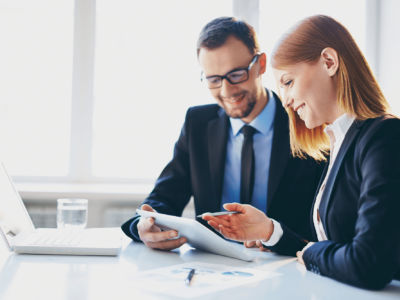
Strategic vs. operational decisions
Within organizations, decisions can be categorized into two types: strategic and operational. Strategic decisions are long-term choices that set the organization’s direction and goals, having a significant impact on its future. These decisions often involve in-depth analysis and planning, such as entering new markets, making major investments, or developing new products.
In contrast, operational decisions are short-term and address the daily management of the organization. These decisions are often urgent and need to be made quickly to ensure smooth operations, such as resolving customer issues, allocating resources, and scheduling work.
Both types of decisions are critical to an organization’s success and can be supported by data, though they require different approaches.
Figure 6: Aspects where time plays a role in strategic and operational decisions include the need to consider long-term vision, thorough planning, sustainable impact, risk assessment, and the long-term implementation of decisions for strategic decisions. In contrast, operational decisions require attention to short-term needs, rapid execution, responsiveness, routine management, and flexibility.
Data for making quick decisions
Data-driven operations can enhance decision-making by ensuring decisions are made at the right times and can be automated where possible. Data analysis uncovers trends and patterns that might elude the human eye, aids in forecasting, and supports simulations to better understand the outcomes of strategic decisions. This shift from broad to targeted micro-decisions helps organizations become more efficient and effective. For further insights, see our page, What is Artificial Intelligence? The Guide to Successful AI & Applications.
Consider an example of an intelligent online bicycle retailer. They leverage data across all aspects of their operations. Operationally, data helps determine staffing levels in the call center, while strategically, it guides major decisions. For instance, the retailer initially sold bikes from other brands but used data to develop their own product. By analyzing website data, they identified customer preferences and created a new bike model—an electric bike with a classic look and luxurious finish—that filled a market gap. This data-driven approach allowed the company to transition from a bike seller to a bike manufacturer.
Legal and ethical considerations in data-driven decisions
When working with data, it’s crucial to address both legal and ethical considerations. Before implementing data-driven solutions, ensure compliance with relevant legal guidelines. The General Data Protection Regulation (GDPR) outlines how to manage personal data, establishing six core principles to protect the privacy of European citizens. It’s vital to verify that your use of personal data aligns with GDPR requirements. More information on the GDPR can be found here.
Ethical issues can be more complex. Handling data responsibly involves acknowledging that, although data is often viewed as objective, it can include subjective elements, especially when AI systems are trained on human-generated data. A notable example is an Amazon algorithm used for resume selection, which systematically favored male resumes due to being trained on historical data dominated by male applicants. This resulted in biased hiring practices and highlighted the challenge of removing such biases from algorithms. As AI systems are based on human-generated data, achieving complete objectivity can be difficult. Thus, careful consideration is necessary to avoid potential discrimination when making decisions based on data.
Summary
Making a decision is a complex process that involves various factors: information, insight, emotions, and empathy. To make the best choice, start by gathering as much knowledge as possible about your options. Apply this knowledge effectively while avoiding distractions from factors that are ultimately irrelevant. Finally, consider the future impact of your decision: what effects will it have on the world and others?
Start making smarter decisions now
Are you interested in working with data to make smarter decisions, but not sure where to start? We have extensive experience implementing data-driven solutions and would be happy to help you with that. Please feel free to contact us.
About Passionned Group
Passionned Group is a leading specialist in data-driven decisions, data analytics and AI. Our passionate and experienced consultants and interim managers help smaller and larger organizations to transform into intelligent, data-driven organizations. Every other year we organize the Dutch BI & Data Science Award™.
Frequently Asked Questions
Why can it sometimes be difficult to make a decision?
Indecision often arises from fear of consequences, an overwhelming number of options, or insufficient information.
Why do I often put off decisions?
Everyone procrastinates occasionally, and it’s often not due to laziness but rather to how you feel about the decision and its expected impact. If you fear negative consequences or feelings after making a choice, procrastination can occur. To overcome this, focus on the purpose of your decision and practice self-forgiveness. Remember, everyone makes mistakes, and if a decision doesn’t turn out as planned, life will go on.
What is decision fatigue?
Decision fatigue occurs when making numerous choices in a day becomes mentally exhausting, similar to how intense physical exercise can be tiring. After taking some time to rest, it becomes easier to make decisions.
What are the consequences of bad decisions?
Bad decisions can lead to negative outcomes but also offer valuable learning experiences and opportunities for improvement. Sometimes, the benefits of a different choice become clear only in hindsight, but this doesn’t diminish the value of the original decision.
What are the benefits of data-driven decisions?
Data-driven decisions offer benefits such as increased accuracy, improved efficiency, better risk management, and a stronger foundation for strategic planning and innovation. Explore all the advantages here.
Can data-driven decisions be completely objective?
While data-driven decisions aim for objectivity, they can still be influenced by biases in the data and models. It’s crucial to continuously check for bias and consider ethical implications.
How can I improve my decision-making skills?
Enhance your decision-making by gathering relevant information, analyzing options critically, and understanding potential impacts. Develop self-awareness to recognize biases, and practice decision-making through simulations or real-life scenarios. Learning from past decisions and seeking feedback can also help refine your approach.