Take Business Intelligence seriously and avoid a false start
In this white paper, the author shows the 101 steps you should take to achieve success with Business Intelligence. After following all the steps, you will have reached the very highest level of maturity in BI. The author is very meticulous by really naming every (small) step. He has more than 20 years of practical experience in the field and teaches at TIAS and at the Passionned Academy. Download the white paper directly here.
After reading the white paper, you will learn that:
- Business Intelligence should always connect to decisions
- Very close involvement of management and users is essential
- In-depth knowledge of the field is crucial for both business and IT
- Several roads lead to Rome, but you cannot skip steps
- Being successful with Business Intelligence is not always easy
Why this white paper?
The author Daan van Beek has more than 20 years of practical experience in Business Intelligence. Many customers recently asked for a practical white paper that describes all the steps in a compact way.
Get serious about BI
Learn in depth about the most essential steps in BI and then test if you are on the right track.
About the author: Daan van Beek MSc
Daan van Beek is ultimately responsible for the Passionned Group, speaks at conferences and also writes books including the well-known Data Science book. Daan also lectures at TIAS and EUR and gives Business Intelligence master classes in India, Singapore, South Africa and New York.
Introduction
Business Intelligence is still often seen as an IT product. But it certainly isn’t. So many report factories are still being rigged up. This is not just a waste of money. After all, who opens and reads all those reports (on a regular basis)? But you don’t dismantle a report factory overnight. It significantly reduces the chances that you can ever again achieve success with BI.
In this white paper, the author describes all the steps needed to achieve (great) success with Business Intelligence.
Reading guide
Before you go through the 101 steps, here are some notes.
- It is a complete list tested by experts with many years of experience in the field. Each step obviously requires a number of smaller steps.
- The steps focus on achieving success with BI as quickly as possible and are not aimed at creating a report factory or compliance reporting.
- For quite a few steps we offer support as consultants. You can always contact us for advice without any obligation, as your situation is likely to be slightly different than usual.
- For a number of steps we also have practical templates available such as the project plan, functional design, KPI’s et cetera. Please contact us for more information.
The scope of BI & definitions
To make Business Intelligence a success, several disciplines in the organization must work closely together. It is a multidisciplinary field. Therefore, we list here three definitions of Business Intelligence from different perspectives:
- BI as a process: BI is the process by which you register, collect, combine and analyze (big) data in a targeted way. You consistently use the resulting (predictive) information and knowledge to make better decisions. The goal: to substantially increase performance at all levels in your company.
- BI as a tool: BI is the collection of tools and IT resources that supports, makes efficient and gives a face to the BI process in organizations. BI technology ideally provides a single source of management information that humans and machines (AI) leverage. See all 25 BI tools.
- BI as a discipline: BI is the set of concepts, processes, strategies, culture, structure, methodologies, standards and IT tools that enable organizations to become more intelligent.
In this white paper, we emphasize the first definition.
We use a fairly strict definition of BI success. Organizations that are successful with BI achieve better results thanks to their Business Intelligence. These include lower work stocks, shorter lead times, higher margins, increased customer satisfaction and a larger market share. BI’s contribution to these result areas is demonstrable.
The 101 steps to Business Intelligence success
Achieving success with Business Intelligence does not come easy. It is not a matter of luck. Rather, it requires a process of meticulous engineering. Step by step, you build and expand success. These are the steps to lasting Business Intelligence success, and this is how you develop an intelligent, data-driven organization:
- Understand BI & data-driven work yourself: make sure you build up ample knowledge and experience with BI dashboards, reports, data warehouses, machine learning, KPIs, decisions, etc.
- Let others understand BI too: make sure that you gather enough supporters within the organization who also have or want to build up that knowledge and experience.
- Create a prototype: download one of the BI tools, collect relevant data and build your first BI dashboard with interactive capabilities. Go public with the data and your dashboard.
- Increase awareness: with all the knowledge that you and your colleagues now have (and the prototype) you are going to increase awareness of the possibilities and benefits of Business Intelligence. Especially among decision makers in the operational process and management. Consider inspiration sessions, interviews, demos of the prototype and working workshops.
- Assemble a BI working group: identify all stakeholders and future users in the organization now and select a number of people from that group for the BI working group. Get Finance & control and IT on board and able to think along.
- Scan your IT vendor contracts: in some contracts, vendors prohibit direct access to your own data. This can be a major roadblock to the further development of Business Intelligence. Make sure the barriers to data access are removed.
- Write a vision document: have the working group develop a common vision for the overall field. Make sure that the vision comes between the ears of all members of the working group (with concrete applications and all) and that they can explain and propagate it themselves.
- Management approval: present the vision to management and other important stakeholders. Make sure that management can give approval to the vision document.
- Map decisions integrally: using the decision diagram, start mapping all the important (operational) decisions in the primary process. These are your use cases. Focus on the outline here but for each decision try to work out all the cubes as best as you can.
- Define key KPIs: after you have a good understanding of the key decisions, you can easily retrieve and further define the KPIs. Align these with the Finance & Control team.
- Prioritize decisions and KPIs: together with key stakeholders and management, you are going to prioritize decisions and KPIs. Ideally, you are going to optimize those decisions that also contribute (significantly) to the business strategy.
- Select the most important decision and KPIs: following the previous step, you let the board make a decision on which decision should be supported first by a Business Intelligence dashboard or BI system.
- The board appoints a delegated principal: the board has a delegated principal represent itself operationally for that specific decision. Who is also the Product Owner BI.
- Assemble a project team: in consultation with the Product Owner, assemble a project team that includes a data engineer, a BI consultant / dashboard developer / report builder, a data analyst and a data coach. Appoint a project leader.
- Write the business case including project plan: describe and visualize what you are going to do (activities), with whom (roles), how much time it will take (WBS), what are the goals that you want to achieve, what risks there are and what measures to take against them.
- Organize a kick-off: with the project team and key stakeholders, organize a kick-off meeting so that everyone is up to date on the latest developments. Present the project plan, ask for feedback.
- Get the project plan approved: IT, Finance & Control and the delegated client should approve the project plan.
- Communicate the project to the organization: have the Product Owner communicate the project to the organization/department. Focus on those directly involved but don’t forget to keep key stakeholders in the loop.
- Create a functional design: describe what you are going to achieve in as much detail as possible. Don’t forget to define the KPIs and PIs including what data sources you need to calculate them.
- Create a dashboard mock-up: sketch in broad outline what the future dashboard might look like. This is part of your functional design.
- Sketch a dimensional model: now describe what you are going to achieve in a dimensional model with measurement values, dimensions including hierarchies, and any linking tables and filters. This model is part of your functional design.
- Make the design AVG-proof: if you have to deal with the processing of (sensitive) personal data, you have to seal off a number of things in your functional design. Privacy by design.
- Get the functional design approved: ask for feedback from the Product Owner and Finance & Control and get them to approve the design.
- Conduct a data quality review: poor data quality should be known to all project team members and the Product Owner. Escalate major problems through the Product Owner to the process owner. If necessary, start a parallel improvement project to raise data quality.
- Outline the project initial architecture: name all relevant principles for the project initial architecture and describe the components needed to achieve the project result. Only in follow-up projects you should start thinking about a complete BI architecture.
- Get the architecture agreed upon: Have IT together with the Product Owner approve the architecture. This will prevent problems later on.
- Choose the right BI tooling: Don’t be overwhelmed by the dominant market position of Microsoft Power BI, but choose the tool or BI platform that best fits your organization’s needs and requirements. Check out our BI buyer’s guide here [year].
- Organize a button training: Organize a button training for the BI tool for your BI team, a few people from IT and for some future users.
- Visit the client: book an informal meeting with the client (board member) and tell them what you have encountered so far in terms of resistance, enthusiasm, setbacks and windfalls. Do this together with the Product Owner.
- Unlock the data sources: let IT, under the direction of the data engineer, unlock the data sources. At least make sure you have a copy of the tables you think you need on another server.
- Provide daily updates: idem, make sure overnight the (changed) data of the previous day is ready. Real-time updates are usually a bridge too far at this stage unless the business case clearly demonstrates that this is absolutely necessary.
- Explore the data: have the data engineer explore the data together with the data analyst. This way you quickly find out where possible problems may arise in terms of data quality, data linking, etc.
- Model the data: now the data engineer can go all out on your data. They are going to screw together a data model that is as robust and future-proof as possible. But for now with the scope being the current decision. In this step, the data model for the customer and product dimension must take into account a larger scope.
- Transform the data: after the updates come in, the data engineer ensures through ETL processes that the data model is updated every night. You also add important formulas for calculating KPIs.
- Test the data model: have a future domain expert with SQL knowledge and experience (or an external data engineer) review and test the data model.
- Process the feedback: incorporate any omissions in the data model and other feedback from the test into the data model.
- Design the dashboard & visualizations: now the data analyst / BI consultant can get to work retrieving the right data from the data model to visualize the KPIs on a dashboard.
- Add interactive elements: make the Business Intelligence dashboard interactive including drill-down capabilities so users can browse and analyze the data.
- Add reports for details: develop detail reports that allow users to take appropriate actions. For example, a report that shows items below the minimum stock level.
- Present the dashboard: host a meeting where you show and explain the dashboard to users. Ask for feedback. Also show what the back end of a dashboard entails so they understand that it is not all simple.
- Have the dashboard validated: have some (critical) users validate the dashboard. Are the numbers correct? Is the design logical? Is it easy to use? Etc.
- Keep IT in the loop: make sure you keep IT regularly (and early) informed of developments. After all, they need to start managing the data model and dashboard.
- Create a production environment: in consultation with the data engineer and the BI consultant, IT will ensure that a production environment is created. From here, users can access their dashboard daily/weekly and analyze the data.
- Set up authorizations: each user has a role in the business process. Set the authorizations depending on someone’s role. This way, users get to see directly what they are responsible for and no one sees unauthorized data.
- Create a manual: document the desired usage in a compact manual (ideally online) or video instruction. Make sure that in this manual the definitions of the KPIs on the dashboard can also be consulted.
- Set up a service desk: under the direction of IT, set up a service desk where users can go when the dashboard does not work (as desired).
- Put the first dashboard live: process the feedback from users and IT and package the dashboard and data model and implement it all on the production environment. Adjust the manual if necessary.
- Give all users button training: take your users through all the possibilities of the dashboard and the possible data analyses that can be performed. Differentiate here between managers (decision makers) and data analysts.
- Communicate the dashboard and data model: make sure that all users, stakeholders and management are informed about the dashboard going live and its purpose.
- Be wary of criticism and resistance: with BI, the way that decisions are made in your organization will be more transparent. Don’t expect everyone to be waiting for this. Middle management in particular sometimes cannot handle this transition.
- Ensure support from the management (again): you need a lot of support from the management (they must be squarely behind you) otherwise some middle management people will downsize or even sabotage your project.
- Organize a party: this great moment should not be forgotten soon. Therefore, organize a party for all involved and stakeholders. Celebrate the going live of the first dashboard.
- Management on the soapbox: during the first act of the party, have a board member and someone from operations say why the dashboard is important: much better decisions through insight and data analysis. And not to maintain a culture of accountability.
- Organize training in data-driven working: perhaps the Achilles’ heel of the project is training and coaching the managers. They, along with the data analysts, must be brought into a natural flow to make data-driven decisions from now on. Data literacy must continue to rise. Request a quote here.
- Organize regular data sessions: either individually or in groups organize weekly or monthly data sessions where you link continuous improvement to the dashboards with KPIs and data analysis. Managers and team leaders should know all about PDCA and data-driven working.
- Link findings back to client: together with the Product Owner, engage with the client and link back your findings so far. Where are things going well? Where is it going less well? How to adjust? What do you now expect from the client?
- Improve the dashboard: collect all feedback from the users and other stakeholders. Incorporate that into a modified data model and dashboard. Make this a continuous process in which the Product Owner directs.
- Appoint data coaches: the data coach helps decision makers in the organization make data-driven decisions on a daily and weekly basis. A data coach also sometimes participates in meetings where decisions are made.
- Limit time and budget: let all of the above cost no more than 70,000 euros and take less than 4 months of time. These are averages and the actual cost depends on the amount of users, the amount of data and the complexity of the decisions and the organization.
- Create a lessons learned document: from all the previous steps, you have learned a lot. Together with the Product Owners, draft a document summarizing the lessons learned.
- Repeat from step 11: make another high-priority decision and then go through the necessary steps, keeping the lessons learned in mind. Do this in half the time and for half the previous budget. You will be able to skip steps. That way you won’t have to select a BI tool or adjust your architecture every time.
- Create data quality dashboards: the more data-driven decisions you start making, the greater the importance of clean, reliable data becomes. Therefore, start developing dashboards for each domain that allow process owners to monitor and analyze data quality.
- Appoint data stewards: the data steward works on behalf of the process owner to operationally resolve data quality issues. He or she also describes the key data of his or her domain and how to improve data stewardship.
- Jump from project to program: the time of separate projects is now over and you will work with the management to create a program “Data-driven working”. So there will be an overarching program for all BI projects.
- Transform the team to a department: the project team also needs an upgrade and a permanent place in the organization. Set up a BICC or data lab. Make sure there is a healthy mix of techies, domain experts, analysts and social animals.
- Attend a Big Data or Data Science training: bring yourself and some colleagues up to speed on the specific capabilities of Big Data, machine learning and AI.
- Develop an enterprise-wide data architecture: after a number of successful BI projects, your architecture is going to squeak and creak. Therefore, give your architecture an upgrade as well; first, design the new architecture on paper. Keep in mind the storage of Big Data and machine learning. This architecture must be scalable down the chain and be able to process data and more complex analysis super fast.
- Question the current tooling: previously you chose a specific BI tool but is it still suitable for the new architecture? This is where you revisit which BI tool or platform best suits your situation and organization.
- Agree on a new architecture with stakeholders: discuss the new architecture extensively with all stakeholders. Map out the impact in terms of budget and stability of the existing IT. Dare to go back on earlier choices.
- Monitor the use of BI: see how often users switch on BI. Talk to them when they use it frequently, but also when it is below expectations. You will learn from this.
- Ensure that data-driven working is the norm: before implementing the new architecture, you need to be sure that all layers of the organization are now working largely data-driven. Therefore, present the new organization and architecture to a large audience and gather feedback.
- Sharpen the previously established vision: update the vision document to include machine learning and present it to management, key stakeholders and the various Product Owners, among others.
- Create a BI roadmap for the next 3 years: in a BI roadmap address all BI needs of the organization (dashboarding, reports, downloads, external reporting, analytics, etc.) for the next few years including Big Data and machine learning applications.
- Present the BI roadmap and request budget: discuss the roadmap with all stakeholders and gather feedback. Adjust the roadmap, resolve any disputes. Make sure you get sufficient budgets for all items in the BI roadmap.
- Migrate the dashboards and reports: you migrate the previously built dashboards and reports into the new data architecture. You do this with common sense so that you don’t transfer dashboards and reports that are no longer relevant.
- Replenish your BI team: at this stage of BI maturity, you not only need more people but also different expertise. More expert knowledge of new technology is needed and at the same time you need more domain knowledge. Change management expertise and experience is also very important.
- Start experimenting with machine learning: explore for yourself where machine learning can make a difference in your organization. Temporarily hire an experienced data scientist to help you get started. As a starting point, always take decisions in your organization, focus first on assessment processes in operations. For example, accepting a policy, appraising property or granting a license.
- Select a language or tool for machine learning: there are several languages and tools that you can use to start developing machine learning algorithms. Consider not only Python and R, but also other libraries and tools such as Scikit Learn, TensorFlow, SAS, etc.
- Develop a prototype machine learning: just like in the beginning with BI dashboarding (what steps you have already taken!) you need a prototype. This is where you go back to farming.
- Name the difference between BI and AI: Business Intelligence is an umbrella term that also includes machine learning and AI. With Artificial Intelligence, you automate the analyses that were previously done by humans. It also allows you to automate possible decisions. Explain this principle to key stakeholders.
- Work out the machine learning applications: based on the decision diagrams drawn up earlier, in workshops with domain experts you will look at how to apply machine learning to the decisions. Pay extra attention to big data and external data, such as weather, demographic data, and how to use machine learning to differentiate decisions in detail. Contact us for an explanation.
- Present and prioritize the machine learning applications: in a working group you will jointly go through and prioritize the machine learning applications under the guidance of the Product Owners and a member of the management. As with dashboarding, you should select one decision at the beginning that you want to apply machine learning to.
- Develop a vision for AI: complete the aforementioned vision document with all the possibilities that exist for machine learning. In doing so, also consider: the more algorithms you develop and implement, the fewer reports and dashboards are needed. And also fewer managers.
- Explore the social impact: the organization can become even flatter through successful AI and basically fewer people are needed to do the same work. Get the implications clear, discuss them with Product Owners and management.
- Make machine learning AVG-proof: these days there is a wind blowing through AI-land where you have to be very careful with personal data and algorithms. And rightly so. Make sure your algorithms stay within the legal frameworks.
- Don’t forget AI ethics: all kinds of ethical dilemmas are around the corner if you want to apply Artificial Intelligence. For example, as an insurer, are you allowed to use AI at the gate to select for possible profitability of an insured? Discuss AI ethics with your key stakeholders and formulate the limits or substantiate AI applications with arguments as to why it is ethical.
- Raise additional budget for AI: You will likely need additional budget to successfully implement AI. Work with the Product Owner to establish a business case for this particular AI application.
- Explore your data needed for AI: before you use machine learning to support or automate a decision, you need to know the data very well. Bad data is disastrous for AI. With a BI dashboard or report, you can still notice bad data yourself but not with a machine learning model.
- Determine a dataset for training: to develop a machine learning model, you should train the algorithm with a representative dataset. It should be free of biases. Don’t stare blindly at your own data. Nowadays you can also buy datasets for training algorithms.
- Have your model validated: together with domain experts and a machine learning engineer you will validate the model. Observe what happens under the hood (as far as you can still understand it) and what outcomes does the model generate given the input.
- Fine grind the model: based on the results from the previous step, you are going to fine grind the model and shorten it if necessary. Make sure that the model performs just right for the purpose. Sometimes a 25% accuracy is enough; the model performs better than a human can do. But for medical assessments, you need much higher accuracy.
- Set up a production environment for AI: as with dashboards and reports, for machine learning you need a production environment on which to run the machine learning models.
- Take the model into production: now the machine learning model is ready to actually start making an assessment or prediction based on new input. You have reached a fantastic milestone because few AI applications make it to the finish line.
- Evaluate performance: be sure to monitor the model’s performance first daily and later weekly. Stay with it the first time and see what happens. Provide a backup system.
- Communicate success: is the performance of the model good enough? Then communicate the success with the organization.
- Organize an AI party: successes should be celebrated together, and this success certainly deserves a party. Invite everyone involved in the success and thank everyone for their efforts and results.
- Determine lessons learned: together with the team, determine what lessons can be learned from the first AI implementation. Summarize them on an A4 sheet.
- Link the AI findings back to client: together with the Product Owner, talk to the client and link back the AI findings so far.
- Repeat step 81: now start automating other decisions with machine learning. Sharpen your vision for AI.
- Define the new organization: all key decisions are now supported with Business Intelligence dashboards, reports or machine learning. Do you need more or fewer employees now? Where can you downsize and where do you need to axe? Together with the board and management, define what the organization will look like in the future. Make a social plan.
- Sign up for the Dutch BI & Data Science Award: every other year we organize this award for the Smartest Organization in the Netherlands where data-driven working is the norm.
Maybe you had already noticed. During these 101 steps, you actually went through 3 phases. First you focus on Business Intelligence dashboards, data-driven work and decisions. Next comes a phase of restructuring with building a solid data architecture and migration. In the final phase, you implement machine learning.
Put yourself in a higher gear
Do you also want to complete the above steps faster and get faster returns on BI? Then invest in one of the products below.
The BI & Analytics Guide™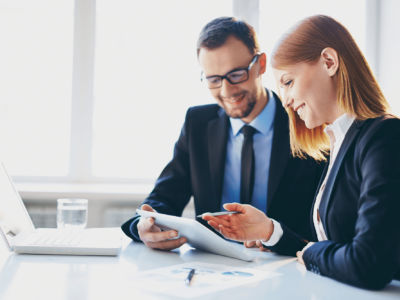
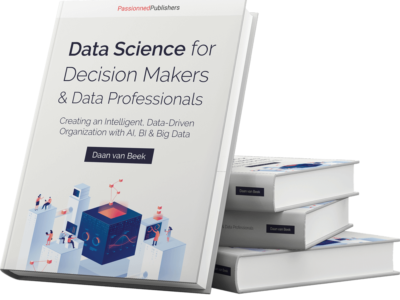