1. Data quality and consistency
Poor data quality is often the biggest hurdle. If the data is not accurate, consistent and current, the BI system will produce unreliable results. Consider duplicate customers or employees, product coding that does not match or address data that is not up-to-date. Users will not trust a KPI dashboard or report when results are unreliable, and they will abandon BI. Delve into how to measure and improve data quality here.
2. Integrating data
Integrating data from different sources, systems and formats is complex. Disparate systems, legacy platforms and different data structures can be a major challenge for data consolidation. Big data also adds complexity to data integration because it is bulky, complex in structure or a combination of the two. Vendors of source applications or systems may not want to release the data or metadata (for nothing). Or dashboard builders may try to solve everything with front-end tools (Power BI, Tableau, Qlik Sense) without thinking about a solid data infrastructure. Read more about data integration with a data warehouse here.
3. Employee resistance
Employees may consciously or unconsciously resist change out of fear of the unknown, anxiety about the increase in transparency or concerns about job security. Fostering a data-driven culture requires active management involvement (leading by example and having a clear vision) and effective change management strategies. Make sure you base Business Intelligence on the decisions the user makes or needs to make. Then you stay close to their perceptions and the likelihood of resistance decreases anyway.
4. Lack of skilled personnel
BI projects require specific skills in data science, analysis, data engineering and more. Finding or training the right talent can be quite a challenge. Also, many (starting) BI consultants are still very inexperienced when it comes to the business and change management side of Business Intelligence. As a result, it will become an IT party and the system will not be used. To find good staff you can use our interim management services.
5. High costs
BI solutions can be expensive. This is true for smaller companies, SMEs as well as larger enterprises or organizations. This includes the cost of software, hardware, training and personnel. Unfortunately, most of the money is still invested in BI solutions that don’t deliver anything substantial, except that you end up with a large report factory or a battery of dashboards. Before you start building a report or dashboard, be sure that it’s really going to add value for the user. Make sure the information is actionable and the underlying data is suitable for interactive analysis. Read our blog to ensure you’re building only smart dashboards.
6. Complexity of tools
While there are many BI tools available, choosing the right tool for the specific needs of the organization is crucial. Some tools may be too complex for the intended users. And many of the Business Intelligence tools contain untold amounts of functionality that most users will probably never need. Don’t look blindly to the popularity of the market leader(s) because you may be disappointed. Prepare a tool selection well with our BI & Analytics Guide 2024.
7. Understanding requirements
Defining clear business objectives and translating them into BI requirements can be difficult. Often, companies are not even sure what they want until they see the first results. In addition, the BI department is often set up on the principle “you ask, we run” and then the results are thrown over the proverbial wall. Understanding user requirements does not start with taking stock of the user’s requirements themselves but with understanding the business, its strategy, its processes and the decisions that need to be made data-driven going forward.
The Business Intelligence book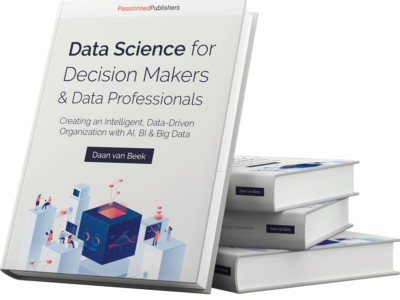
8. Security issues
It is essential that sensitive business data be adequately protected. This means not only protecting against external threats, but also setting authorizations correctly. But perhaps even more important is a culture in which people view (personal) data as a treasured asset and defend it tooth and nail against misuse. Data breaches can also occur (unnoticed), for example, by users exporting data to Excel and storing those files locally. Shield manual exporting as much as possible and make sure the BI tooling does not run locally as an application but in the browser. This already includes standard security protocols that provide a higher level of security.
9. Scalability issues
As a business grows, so does the volume of data and so do the analytical needs. A BI solution that works today may not meet the needs of the business and those of its users tomorrow. Ensuring that the chosen system is scalable is crucial. However, this is easier said than done because needs in Business Intelligence tend to be fluid. That is, the real needs may not emerge until you have been able to gain experience with the BI tools and data analysis capabilities for some time. In any case, make sure you build BI in the cloud, at least then you will have the tools at hand to scale up. But data modeling, indexing and partitioning should also be well thought out and responsive to all future opportunities and challenges.
10. Time to value
Long implementation cycles can make stakeholders impatient and even unhappy. Quick wins can help build confidence, but it is essential to balance speed and quality. Working under architecture is a must but even more important is to design your data infrastructure based on the business issues and not on the data sources as is often done in a Data Vault project. Don’t be fooled by tall tales because the time to value is just a lot longer with a Data Vault.
11. Maintain Data Governance
Establishing a clear data management framework ensures that data is handled appropriately throughout its lifecycle. This includes defining data ownership, lineage, quality standards, privacy, security and more. Data Governance is not just a set of rules and agreements to be followed but it encompasses a whole philosophy that should prepare the entire organization for a data-driven future. Then there is no more need for controlling or enforcing as it gets into the DNA of all levels and disciplines of the organization. Everyone understands the value of good data and then acts accordingly.
12. Keeping up with new technology
The world of Business Intelligence is constantly evolving with emerging technologies such as generative AI, machine learning, new API platforms and advanced analytics. Keeping the BI system up-to-date and integrating new technologies can be a challenge. Of course, you don’t always have to be at the forefront because then you run a greater chance that the teething problems won’t be out yet. In any case, when selecting new technology, always pay attention to interoperability (data interchangeability), scalability and performance.
13. Performance problems
As the amount of data increases, BI systems can face performance issues that affect the speed of data processing and retrieval. Twenty years ago, we thought performance problems would resolve themselves as computing power doubled every two years. But as it turned out, the size of the data also doubled and the complexity of the analysis issues went up as well. The consensus now is that eventually quantum computers are really going to provide a solution but until then we have to settle for cloud platforms, indexing, keeping tables as narrow as possible, partitioning, aggregation, reusing and sometimes it is also necessary to store data twice.
14. Complexity of ETL
The biggest investments go into rigging a robust ETL process. Think of your data sources as crude oil of all kinds and qualities that you have to refine to get to a good end product: diesel, gasoline, kerosene et cetera. You can think of the refinery as the ETL process, which is complex because you have to go from unstandardized to standardized. In addition, ETL is complex because in that process of standardization you have to consider data privacy, security, data quality and performance. With real-time analytics solutions, the complexity can increase even further.
15. The leap to (generative) AI
How difficult is it to jump from reports and dashboard to AI? That in itself is not very difficult, you pull out an algorithm quickly these days, yet in this day and age this is perhaps the biggest hurdle. The challenges that come with reports and dashboards become at least twice as big with AI because it is surrounded by mystique and many users will have great difficulty trusting the outcomes of machine learning. Often also because it sometimes goes completely against intuition. In addition, the challenges around ethics, data quality, privacy laws and explainability are also not insignificant. But don’t be discouraged, AI starts with experimentation so you can gain experience. With sufficient knowledge and experience, you can work on some successful implementations of machine learning. After this, you start thinking about a company-wide AI policy, for example, formulating an AI-first strategy. With AI, always take the decisions in your organization (primary process) as a starting point. In an interactive session of one half day we can help you fully take the next step into AI. Contact us here.
Summary: identify, anticipate and mitigate risks
For a successful BI implementation, it is essential to anticipate these obstacles and develop strategies to proactively address them. A phased approach, starting with a pilot, can help identify potential obstacles early and establish risk mitigation plans. Take the bull by the horns and create a plan or policy with stakeholders. But perhaps most importantly, ensure that the project team and future users have a razor-sharp understanding of how Business Intelligence can contribute to better decisions and performance. See also our new white paper “The 101 Steps to BI Success“.
What are your biggest obstacles? Feel free to respond to this article and describe your obstacle and approach (or tips) to overcome that obstacle. That way we can learn from and with each other.